Rohit Choudhary on Transforming Data Challenges into Opportunities
(Music) Hi, welcome to Data Forward, the podcast for enterprise data and AI. I'm joined here by Rohit, our CEO. I'm Amy Kyleen, I'm the Chief of Staff at Excel Data and we're excited to talk today about why we founded this podcast and some important trends in the industry. Okay, Rohit, let's start out by talking about some of the trends that you're seeing in enterprise data.
Well, a few trends, Amy. So first of all, thank you for having me on this and on the pilot episode. I think it's great to be here. I'm sure that this is going to be a great podcast, so all the best with that. Enterprise data challenges, I think the axioms continue to be the same. Volume of data is just going up and up and up. I think more and more data leaders are getting frustrated by the number of technologies that they have to integrate year over year. Their inability to go find quality talent that will allow them to operate with some reliability. Obviously, the change of technology itself, earlier or five years back, we used to only talk about data and analytics. But now we talk about data analytics, data integration, real-time streaming. We're talking about AI. These are all big challenges for the enterprise leaders to solve today. It's also from a technologist's point of view, I can tell you that this is probably the most exciting time for you to be alive as an engineer because there has never been more excitement in the market overall. I'm sure that the next sequence of solutions, the next sequence of problems will be way bigger than what we ever experienced. But hopefully, we'll have better solutions. Has the profile of a data engineer or a chief data officer changed in the past five years and where do you see it going? I think so. You're spot on with that question and I know where you're leading with this. I think the profile of the CDO, the profile of the data engineer, the profile of the average data user in the industry and in the enterprise, that has completely changed.
Back in the day, you used to know a few relational databases, probably about 10 years ago, and you could get by. But today, you need to know relational databases, you need to know about streaming systems, data integration, different kinds of systems, use case specific purpose-built databases that are being used from generating customer analytics to all the way to regulatory reporting.
Guess what? Who's steering all of these efforts? It's either the CDO or the CIO.
The role of the CDO, again, that has gone through a massive transformation. What used to be after the fact, very, very governance-centric, is converting itself into almost like at the beginning of the food chain, looking at how data is being operated, how is it being activated? That is a completely different experience for all the CDOs. I'm sure that this is very common across the industry now, where people are thinking, "Okay, how do we get ahead?" as opposed to coming and solving problems later. Do you see a federation or a democratization of the way data is being used in the enterprise?
It depends upon how the data is serviced to the different functions. The way to think about it is that more and more departments are looking for granular insights. Advertising has its own set of requirements and its own way of looking at the data. Marketing has its own set of ways of looking at data and finance has to be accurate. But what we are seeing greater than ever before is this requirement of a unified enterprise view of the data. At least some ground truths, which is who are our customers, who are our primary suppliers, when are our contracts due?
Are we spending the right amount of money? Should we be spending less money or should we be over-investing? Those at the enterprise level, they are getting more and more standardized, which wasn't the case earlier.
With AI in the mix, what we are also seeing is that there is an intense focus on the ability of the enterprise to exercise control on that data, which is what kinds of data will be allowed for training in the models, what kind of inferences should we accept, what kind of inferences should we reject. I think this is going to continue as a trend and this will probably have a snowball effect, but to the benefit of the enterprise. I don't think anybody is losing because of this.
So in some ways, the data has more customers, but the data itself is more centralized.
Yeah, I mean, what you take, if you are in advertising, you take a slice of the enterprise data. If you are in marketing, you take another slice of that data, which gives you a unique perspective from that point of view of the business, but the enterprise data is getting more and more centralized. The access is on a need-to-know basis because obviously you don't want all your sensitive data going into field and operations teams. You want to have absolute control over who gets to see that access, but obviously there's a whole big pie and you're slicing it and giving it away.
You touched on this earlier, but what in your view doesn't mean to be AI-ready for an enterprise?
Oh, it means a lot of things. And the way that it starts is defining what's the enterprise value that you're looking to create. What kind of experiences are you trying to improve? Because all the things that AI can do as of now, and we haven't seen new use cases emerge just because of technology improving, is already being done less efficiently today in the enterprise. So whether it is about improving the top line through additional revenues coming in by building revenue, generating new use cases, or improving the bottom line, which is by improving efficiency of the number of people who work in the enterprise, or whether it is for improving C-side because your customers are getting better service from the enterprise, those are, I would say, three big categories of where AI can be implemented. But the first is the anchor is that, okay, what are we trying to do? I think companies which are getting successful with AI implementations, they're able to figure out what is the primary use case. Consequent to that, you're trying to find out, okay, where are these data sources present? And you're trying to integrate all of that. And the way that I have simply understood it is that there are facts and there is context. Facts is in the structured and semi-structured data that exists in the enterprise.
Context is what is stored inside the unstructured data stores, which is Wikipedia's, Mail's, You know, OneDrive, Dropbox's, etc., etc. And when you marry these two together, it almost becomes like a formidable advantage because not only are you telling facts accurately, you're also telling the story and the context that either customers or partners or management or employees need to hear. And I think that is going to be a very powerful combination. Now, in order to do that, you have to have, you know, a bedrock of solid technology, a ground truth which is available in data, and a mechanism of bringing it all together with the right level of security, privacy, governance and control. So it's a different world altogether. Do you believe that structured data and the importance of structured data will go away over time? It's impossible. Structured data is the best inference that you can get. So if you're reading through the balance sheet of Google or Cisco or, you know, Adobe, you can figure out whether the company's strategy is right or wrong if you're a financial analyst. You may not have the qualitative aspect of it, but it is the final summary, which is in many ways the lagging indicator. But you cannot, you know, let's play out five years from now. And the AI halves have it. In that world, too, facts will continue to retain their predominance because the revenue numbers have to be accurate. We will have a fixed number of employees. There will be X number of customers and Y number of suppliers. And so these things won't change. So the importance of facts, if anything, it keeps going higher and higher because the more accurate your facts are, the better your business will be. The better context you'll be able to generate on top of, you know, the factual data. I've also heard you describe structured data as the most efficient way to represent, you know, a broader picture. It is a summarization. Just think of any board meeting that you've gone to or any exchange of information where you had to buy a service or a product. You know, once you're satisfied with the quality and what you need, then the next or the most important thing in your purchase decision is at what price point? So price is almost like a true indicator of value in that case. And I think that will continue to be true. Makes sense.
So you talked about compliance, security and control.
Can you tell us a little bit about how you came up with the idea of data observability and how that fits into that framework? I think so. I think what happened, you know, I think I've mentioned this story many, many times to you and to many others. I was an application engineer and I found out that, you know, the tools for post-production application deployment were very mature. And then I became a data engineer and I found out that data engineering does not have those tools that application engineers forever had access to. And then we started looking at the growth of data, the deployment of big data platforms inside multiple enterprises. And we figured out that this is a unique opportunity for us to build a large company where data applications and data products will become mission critical for the enterprise. And in order for large enterprises or small enterprise to be successful with their data strategy, they needed visibility into how good their data is, how effectively are they able to provide complete data to the different kinds of stakeholders inside the company. And we felt that observability just as a systems engineering concept is as applicable to data products as it was in the application world.
Now, it continues to sort of, you know, just enrich and expand into newer spaces. Because what we also found out was that the amount of metadata that we have already collected, which includes, you know, user operations, SQLs, tables, joins, notebooks, and all the other things that people are deploying, not just in one technology source, but in multiple technologies, within a multi-cloud, multi-technology world, we are finding that we have all the insights to answer more complex questions, which involve, let's say, you know, you could tomorrow ask a question, how much or what percentage of our data as an enterprise leader, you can ask this question and say, what percentage of my data is HIPAA compliant? And we will give you a good answer because of all the metadata that we have, the automated classification that we have, the understanding of the domains that we have built in the last few years. So this ability, this operational view of the data that we have, can be extended to give insights into all the complex regulatory operational revenue generating use cases that people have.
Has it gone to where our vision is as a company? It's a journey. We are getting there, but we are progressing really nicely. That's super interesting. Another huge trend that we've seen, obviously, over the past, you know, 10 plus years is transformation to the cloud. Yeah. Moving to the cloud.
Do you think that all data will move to the cloud? How do you think about where enterprises store data and how CDOs are making those trade-offs today?
I think it is a journey. We've seen some of the leading enterprises move completely to the cloud. And in some cases, we've seen the completion of that transformation happening with a steady-state equilibrium between what stays on-premise and what goes on to the cloud. It is also expected that because of AI, a lot more data will move into the cloud. But there's also the counter possibility that because of AI, a lot of data might end up staying inside the enterprise because of security privacy and governance concerns.
And also because it might be just cost prohibitive to lift and shift all the data onto the cloud.
What we are seeing is that the first inning of the cloud transformation is nearly complete. People have experimented with the cloud. They've incurred the cost. They've done the transformation. And I think a lot of teams have developed the skills and the comfort to deal with cloud technologies. And I think that's a given.
I think what will end up happening is that the availability of high quality of applications and the availability of tools and technologies to manipulate and convert and transform data for their specific purposes will evolve at different velocities for different infrastructures.
And it is fair for us to say that cloud becomes the biggest substrate on which data will lie. Will it contain all data? It's hard to say as of now. But the way we look at it as when we talk to our enterprise, exact sponsors, it's clear that they're taking a very, very long journey. And they're being very thoughtful and mindful about it. And which-- the final angle on this is that they're also looking at it from a regulatory perspective. Because if you're a bank and you're transacting across 150, 200 countries, then every time that you rewrite or rebuild or recreate that application in a new substrate, it has to go through the same regulatory compliance. And that's the hidden cost of transformation, not just technology.
I believe that a lot of new technology is going to be cloud-centric, though. And therefore, all companies will have, by default, a huge presence on the cloud. Will that be 25% in the next five years? We don't know. We just heard from Andy Jesse, I think, last year, that less than 10% of the data is on the cloud right now. So we still have a long way to go. So I want to ask you a little bit more about AI.
Who have you seen that have really identified real use cases and are deriving value from AI in the enterprise today?
So that's a great question. I think it starts with the customer. Because I think a lot of enterprises have gone to the customer satisfaction use cases, serving your customers better. So if you remember, for the last five, seven, 10 years, chatbots have come into existence. So much of the work gets done using chatbot. You need a new phone. You can chat with the bot, but you can also chat with someone. So there's always human in the loop. And I think the customer use cases, they've taken off really, really well. The other place where we have now seen a lot of AI traction is vertically integrated applications. One of our board members brought this up with me, I think, two weeks ago. A few weeks ago, not two weeks ago. And I figured out that the vertically integrated application market, which is supporting cases like use cases such as the medical profession, helping doctors, the legal profession, looking up case law and interpreting some of that, I think that is definitely interesting. And obviously, the most visible one, in addition to all these three use cases, is the whole marketing thing. There's so much of the content which is being generated today is being generated using AI. And not just for what is for public consumption. A lot of internal material is also getting written by that.
The fifth and probably the most common use cases, I think most of the enterprise leaders, CIOs and CDOs included, they've actually enabled all the developers with co-pilot experiences. And those are doing really well.
I don't think this is the end of it, but these are good beginnings for people to go and try the AI technology out and figure out what is the best use case for them. Ourselves have rolled out all of these capabilities. But in addition to that, we've also enabled our products to have AI-first capabilities, which is can we do domain-based classification? Can we create the data quality rules? Can we automatically create thresholds for alerts? And these are super interesting use cases, because what it does for our business is that it allows us to go and activate our products much faster in the enterprise. And I'm sure that in the next couple of years, we'll see tremendous improvement in these capabilities across the board. Did you see this study that AI gave the best recommendations in terms of identifying diseases based on symptoms better than doctors and better than doctors with AI? I'm not surprised.
This was a use case that came to me in 2018 when I figured out that a very popular cancer hospital in India was analyzing all the tumor reports and the tumor x-rays. And they actually fed all of that. And they were able to actually do the aging, the staging, and the grading for the cancer diseases. And they were also able to predict
which patients are more likely to have cancer in the future, and turns out that that prediction is still playing out. So I'm not surprised, because a lot of this has been done, but it's not been done in the way that it is being done right now, which is a huge body of x-rays. If you look at a traditional radiologist, they have tremendous amount of experience by reading and looking at so many x-rays and films. And then they write these reports, which are very, very technical. But the LLMs do get it. They can understand, once you feed them the right level of information, they will be able to predict some of that. Is it surprising?
Maybe, but maybe not so much, because all of this is open to interpretation.
So in this world where the ground is shifting underneath us with AI, what do you think is important?
What are important characteristics of a data leader today?
I think just developing a lot of awareness of this changing technology is super important. If you are an AI leader, you've got to be reading a lot of stuff every week. And a lot of people ask me, oh, but you know what we read last week is no longer relevant this week. And my point is that that is exactly the reason that you want to keep up with the changes and advances that are happening. Models are actually giving out so many better answers. And if you ever want to get into the business of fine tuning the large language models or create your own small language models or run quantized models, you should get familiar with the technology that we are talking about. It was surprising to me when I entered a room and asked, do you know what a model is? And I did not get one straight answer about what it was. What does a neural net really look like? Nobody could answer that question. So I was kind of surprised.
And I think a deeper understanding of this fundamental technology will help for the next five years, but probably even longer.
A lot of things will get abstracted. And that's a given. All technology pieces, the technology's job is to abstract complexity. But it's also interesting at this point in time to take part in the revolution that's happening, to keep it exciting for yourself as opposed to getting disappointed and say, oh, all of this is going to get eaten by AI. No, you can actually be a net participant in that and say, OK, look, I'm going to change the following things by using these disruptive technologies. The eater or the eaten. Yeah, kind of.
So tell people a little bit about why they should tune into Data Forward and what we have coming up. Yeah, I think when we thought about this podcast at Excel Data, we figured that there was not a good data management podcast which was going on. There was not enough information that I felt was available centrally. So when this idea came up from the team, I said, that's absolutely the right thing. There's so many data leaders who are operational by nature, who actually acted on so many of the use cases that Amy and I were talking about right now. And I think this is an exciting place for people to come and learn.
Thanks for listening to the Data Forward podcast. If you enjoyed this episode, please share it with others. Post about it on social media or leave a rating and review. To catch all the latest insider news, be sure to subscribe. And we'll see you next time.
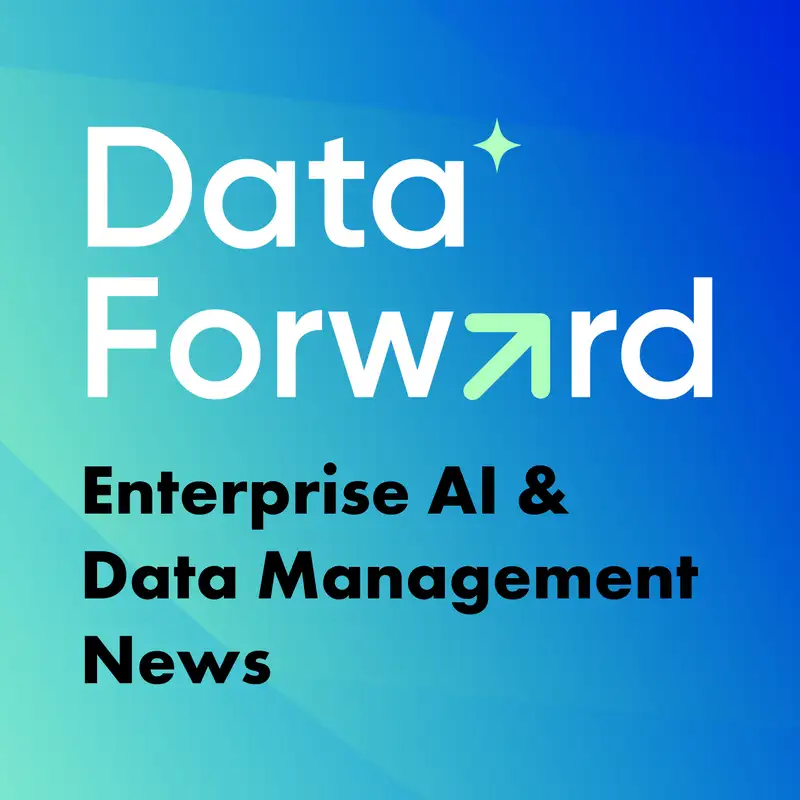